Forests harbor most of Earth’s terrestrial biodiversity and play a critical role in the uptake of carbon dioxide from the atmosphere. Ecosystem services provided by forests underpin an essential defense against the climate and biodiversity crises. However, critical gaps remain in the scientific understanding of the structure and extent of global forests. Because the vast majority of existing data on global forests is derived from low to medium resolution satellite imagery (10 or 30 meters), there is a gap in the scientific understanding of dynamic and more dispersed forest systems such as agroforestry, drylands forests, and alpine forests, which together constitute more than a third of the world’s forests.
Today, Meta and World Resources Institute are launching a global map of tree canopy height at a 1-meter resolution, allowing the detection of single trees at a global scale. In an effort to advance open source forest monitoring, all canopy height data and artificial intelligence models are free and publicly available.
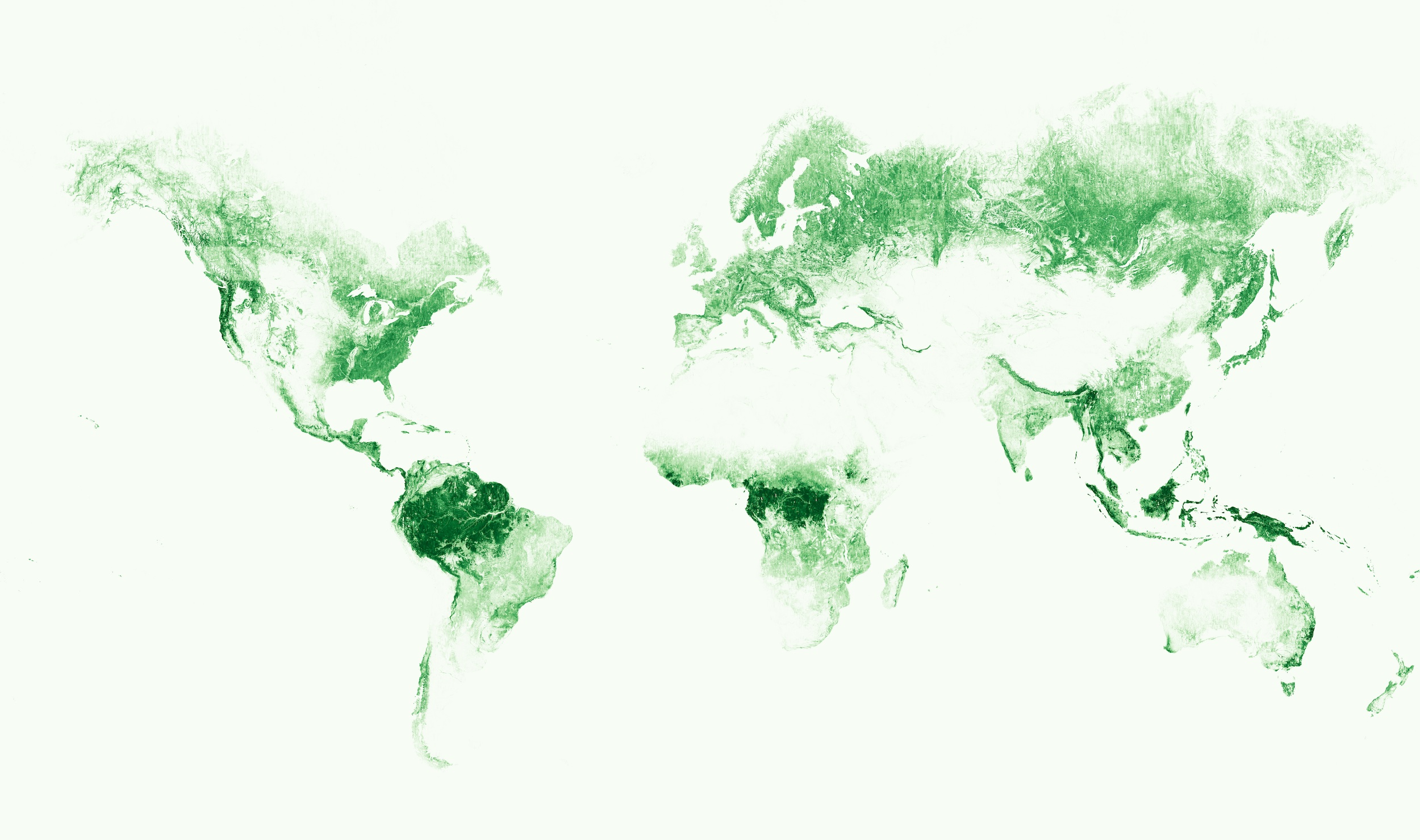
Carbon removal credits require high resolution and large scale mapping
Meta has set a goal of achieving net zero emissions across its value chain in 2030 which will largely be achieved by reducing corporate emissions. Meta will address any residual emissions through carbon removal, including a range of both natural and technological approaches. Forest-based carbon removal and the use of technology to better monitor, report, and verify the carbon sequestration is an essential component of Meta’s carbon removal strategy.
Nature-based carbon removal (including restoration) contributes significantly to the emissions reductions necessary for achieving the goals of the Paris Climate Agreement. Managed forest land has emerged as a major source of carbon credits in the voluntary carbon market and the IPCC considers forest management an important tool to mitigate the impacts of climate change. To manage forests at the scale required to mitigate climate change, it is critical to improve the monitoring and the verification of forest-based carbon credits worldwide, particularly by improving the spatial resolution of forest structure data. Importantly, improving remotely sensed data with artificial intelligence can both help mitigate the gap between reported and measured land use emissions, and enable monitoring across international, national, local, and corporate scales of conservation and restoration projects.
The accelerating pace of breakthroughs in artificial intelligence and foundation models are changing the ways in which we all interact with the world around us. In recent years, mapping of forests through remote sensing has made rapid improvements in terms of scale, resolution and refresh rate (i.e., 1-meter sensitivity as well as being able to detect small changes in canopy height). Deforestation can be monitored with relatively low resolution imagery since a deforestation event often occurs over a large area. Additionally, deforestation typically entails the removal of larger, more mature trees which is often more easily detected in satellite imagery. In contrast, afforestation and reforestation projects present an increased challenge to monitor tree growth, where monitoring of young trees, sparse trees (such as in agroforestry) or small project areas (such as in community-led efforts) require individual tree-scale sensitivity across large areas.
Meta and World Resources Institute believe that democratizing access to artificial intelligence can be an important tool in unlocking finance for and increasing transparency in mitigating and adapting to climate change. For the first time, the compute scale and AI models required for the global processing of high resolution maps are available. We have released both the data and model under licenses permitting commercial use, enabling anyone to build on top of the data to further facilitate accountability and transparency in the carbon market and other conservation and restoration applications.
You can find the data on AWS and Google Earth Engine, the model on GitHub and the paper here.
Details on our approach
Making progress towards this continuously updated map of the Earth’s forests, today we share the canopy height maps we made of the full global landmass1. This dataset creates a global baseline of tree canopy height including individual trees and forests with open canopies. The dataset facilitates global accounting of forest inventory at a high level of detail. Our data finds that more than one-third of the land on earth (50 million km2) has an above 1-meter canopy height, while 35 million km2 have a canopy height greater than 5m.
The dataset analyzes the best available satellite imagery from the years 2009 through 2020. While cloud cover and seasonality impose limitations on the analyzed image dates, eighty percent of the data is produced with imagery from between 2018 and 2020. This baseline can be used as a reference for supplementing field-based measurements of carbon in carbon credit monitoring and verification schema. When newer imagery is available, the publicly shared model can be used to detect change in canopy heights.
To create the maps with reasonable resource usage, we needed to achieve both a globally robust model as well as fast inference. We achieved this by leveraging a state of the art model based on methods developed by AI at Meta Research named DiNOv2. The model was trained on 18 million satellite images (0.5m natural color imagery from Maxar Technologies), encompassing more than a trillion pixels, from across the globe. The AI model allows us to predict canopy height for a given area with a mean absolute error of 2.8m, enabling the detection and measurement of individual trees. In order to expand the applicability of the AI model, we have built it to use both aerial and drone imagery when resampled to the same 0.5m resolution and similar color balance. The details of the model and performance can be found in our recent, peer-reviewed paper.
We use the powerful approach of Self Supervised Learning (SSL) to obtain a globally consistent high resolution foundation model for earth observation. This involved training the DINOv2 model solely from unlabeled satellite images. This approach teaches the AI model to extract general image features without the need for expensive and time consuming labels. The SSL architecture provides a backbone of visual perception which can be used for inference of any type of feature. For our application, we train a canopy height predictor on top of the SSL architecture by utilizing a modest sized sample of LIDAR ground truth data from the United States (the NEON dataset).
DINOv2 has shown to be a very effective foundational model for diverse downstream tasks. For instance, its backbone trained solely on natural images has shown strong generalization for depth estimation, and part matching on art imagery such as drawing and paintings. Similarly, we hope that our released global earth foundational model can be leveraged for other downstream tasks than canopy height estimation, such as tree detection and segmentation.
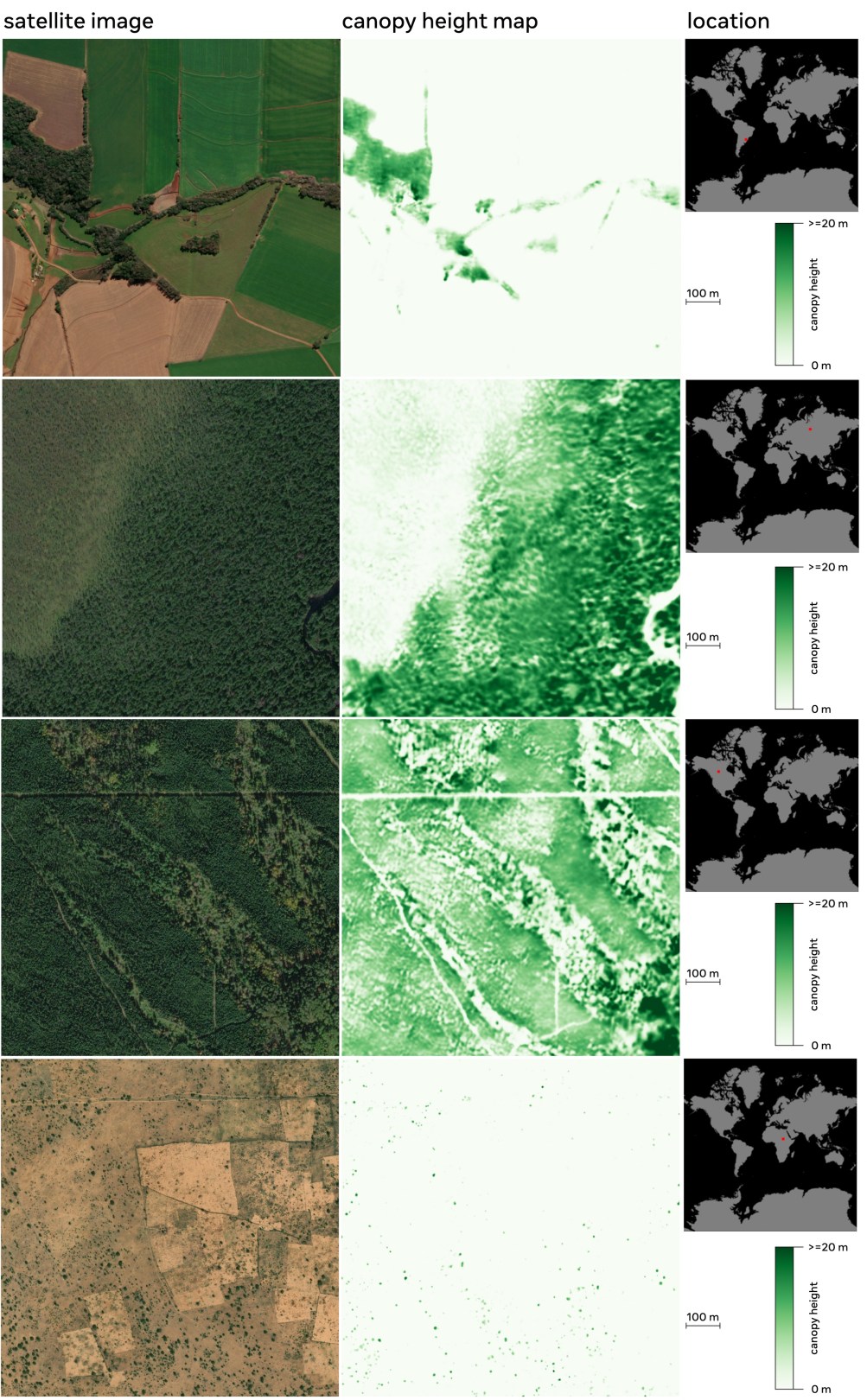
Examples of the canopy height maps on four different continents. The left panel shows the satellite image (from Maxar Technologies), the middle panel shows the predicted canopy height, and a red dot in the right panel indicates the location where the analysis was done. The model generalizes well globally across different ecosystems (see paper for details).
The canopy height map can be used as a starting point to extract above ground biomass and to establish baselines for conservation and restoration projects. World Resources Institute manages TerraFund for AFR100, a partnership that has invested in 192 local nonprofits and enterprises who are restoring Africa’s degraded land. In total, they are restoring more than 29 million trees across tens of thousands of sites. The Land & Carbon Lab at World Resources Institute is utilizing the canopy height data— and the foundational DINO AI model — to connect high resolution satellite data with extensive field-based forest inventory data. This information will allow the initiative’s managers to reward projects that are meeting their targets, identify and provide capacity support to those that are falling behind, and transparently communicate impact.
Conclusion
Forests play a critical role in the overall global carbon market and are essential to protect the natural world in the face of a changing climate. Data is paramount to support the monitoring of forest restoration and conservation efforts, so that the planet’s biodiversity is preserved and climate change may be curbed. We believe that regularly updated maps of canopy height like those we have released today, dramatically improve both forest-based carbon credits and non-credited natural climate solutions. The recent developments in high resolution satellite imagery, artificial intelligence, and computational capability have unlocked the ability to create a global canopy height map at the resolution of single trees on an annual basis, which will greatly benefit the preservation of the Earth’s forests. To maximize the potential of this work, we are complementing our new global data with openly accessible models to enable others to build off of our work.
1 We excluded Antarctica and Greenland from the maps.
News
Get the latest news about our sustainability work, download reports and resources and explore our energy dashboards.
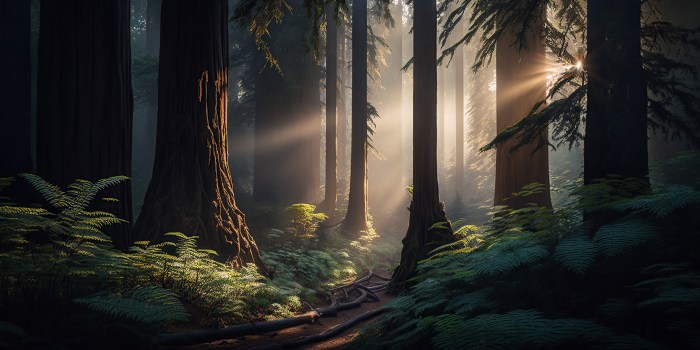
Technology vs. Trees: What The Debate Over Carbon Removal Is Missing
If you pay attention to voluntary carbon markets, particularly if you’ve been working with them for more than a few years like I have, you may have found the past year of seemingly constant media coverage and debate to be rather dizzying.
January 5, 2024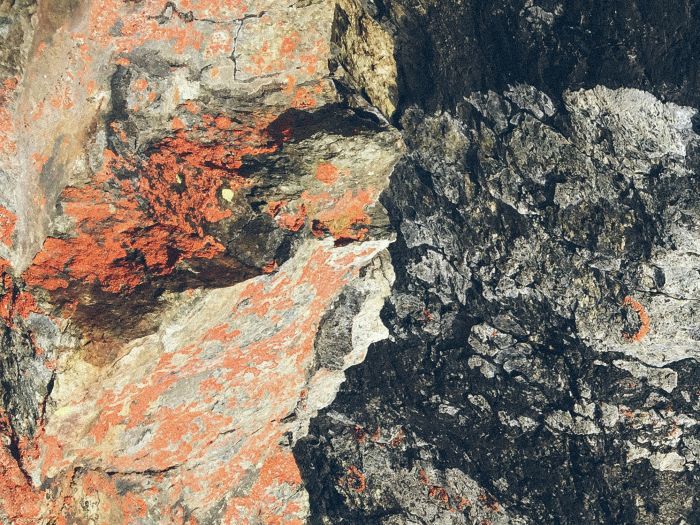
Frontier announces direct air capture (DAC) offtakes purchase on behalf of Founding Members with CarbonCapture Inc. and Heirloom
CarbonCapture and Heirloom will remove a total of 72,000 tons on behalf of Frontier buyers, including Meta, by 2030.
November 16, 2023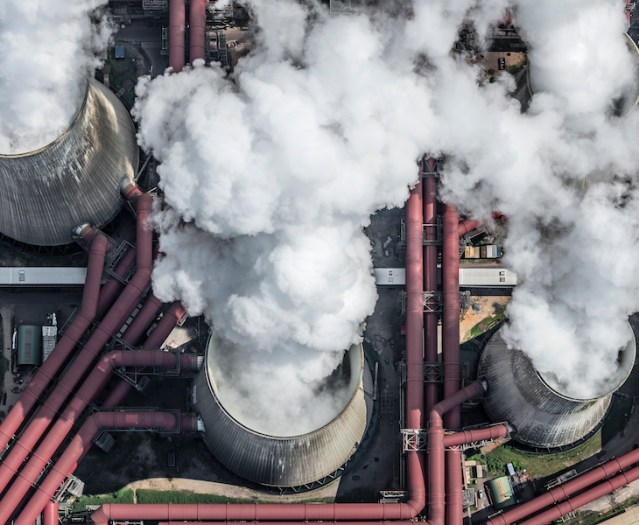
Investing in Value Chain Emissions Reduction Projects
The scope of this RFI is specific to our efforts to invest in value chain emissions reduction projects. As such, we are initiating an RFI for value chain emissions reduction projects so that we can directly connect with entities that are positioned to originate, host, and/or support a value chain emissions reduction project or the increased offtake of low-carbon solutions or materials.
August 1, 2023Focus areas
At Meta, we’re building a better reality at every level of our impact.
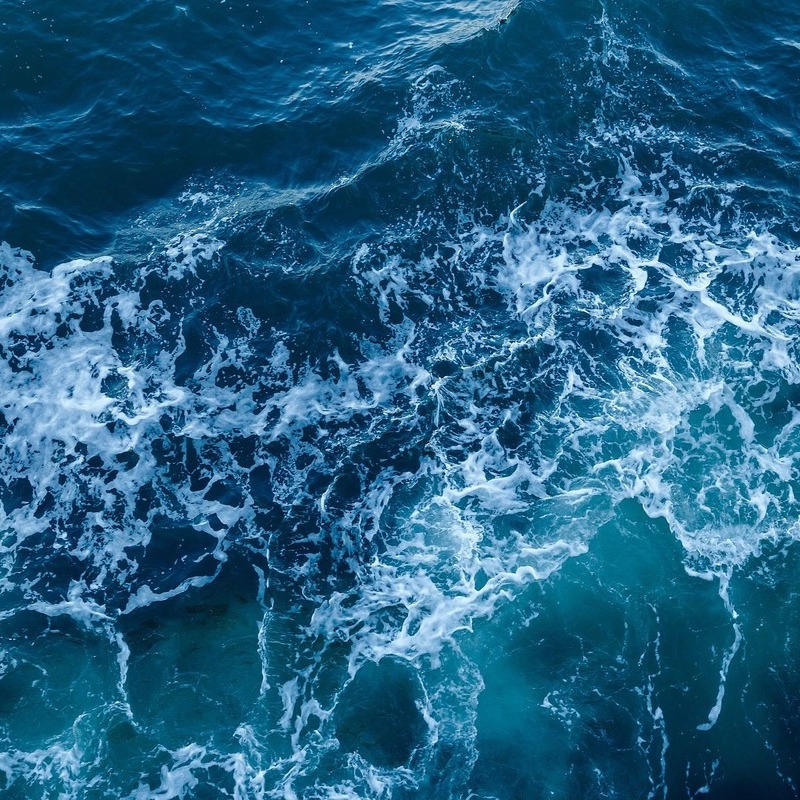
On our planet
Taking daily action toward net zero
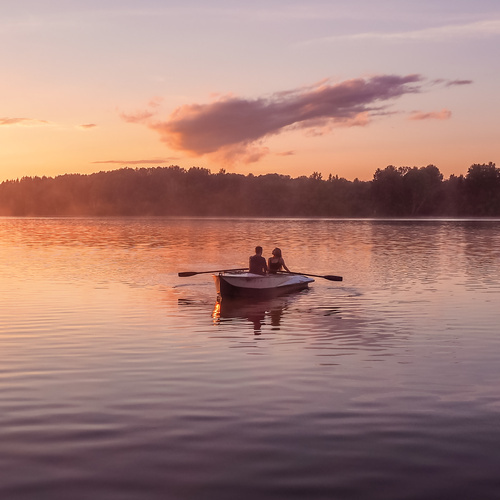
With our resources
Promoting resiliency and restoring loss
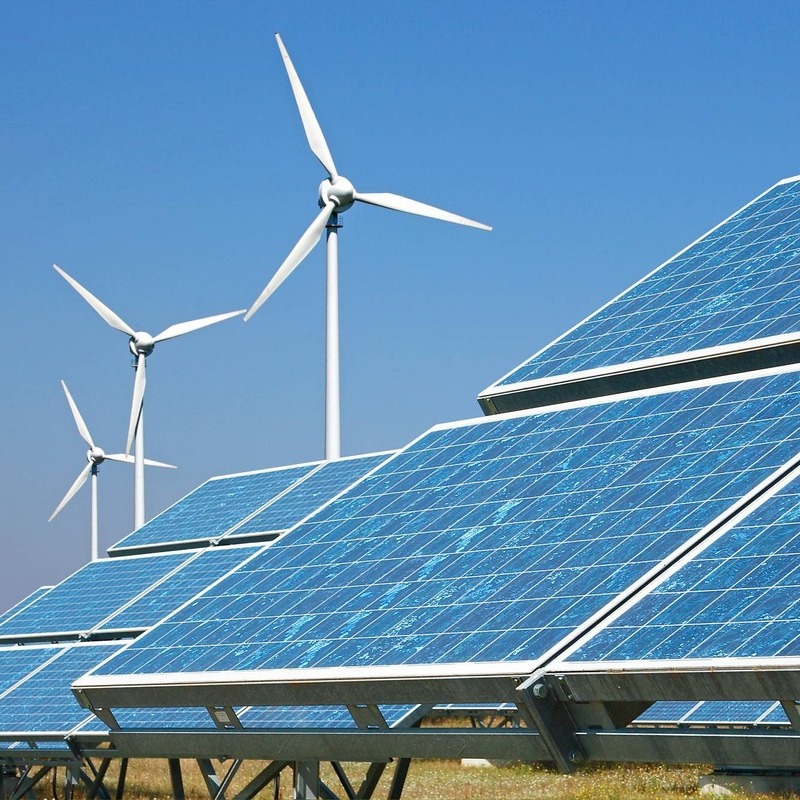
Across our footprint
Driving efficient management of resources
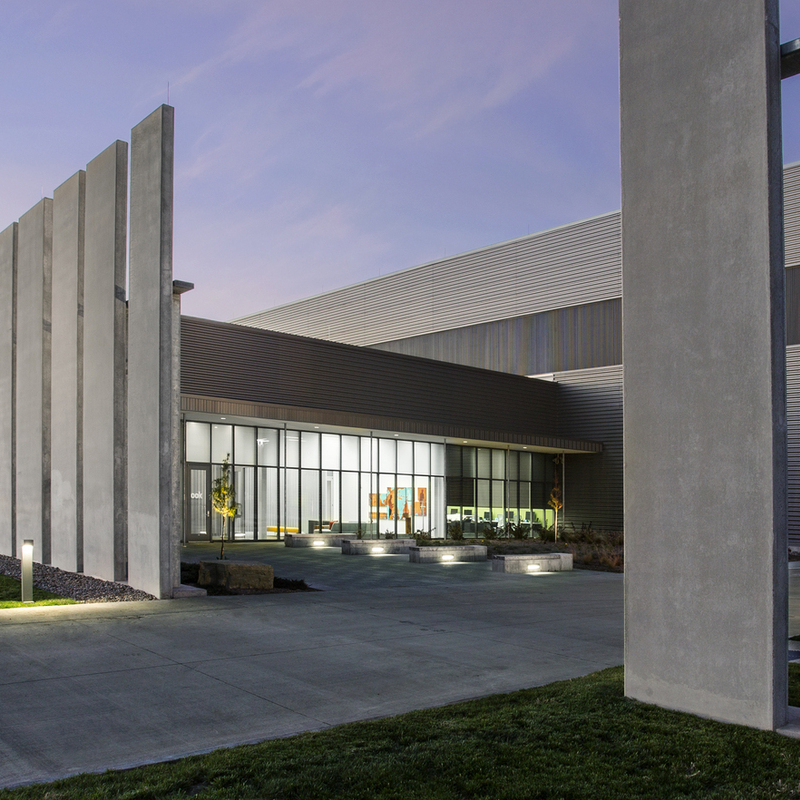
Within our facilities
Meeting the highest standards for sustainable buildings
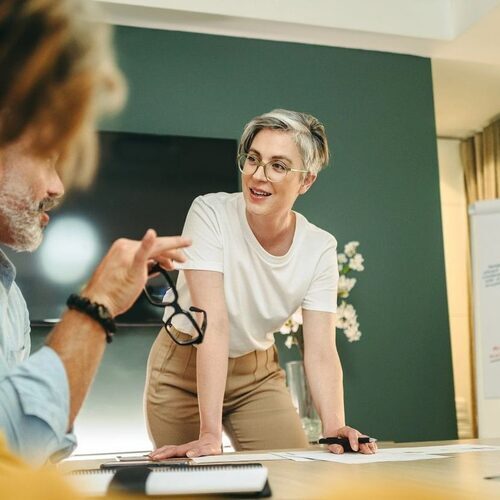
Throughout our business
Working together for the highest outcome
2024 Sustainability Report
Learn more about our progress as we work to achieve net zero emissions across our value chain and become water positive in 2030.
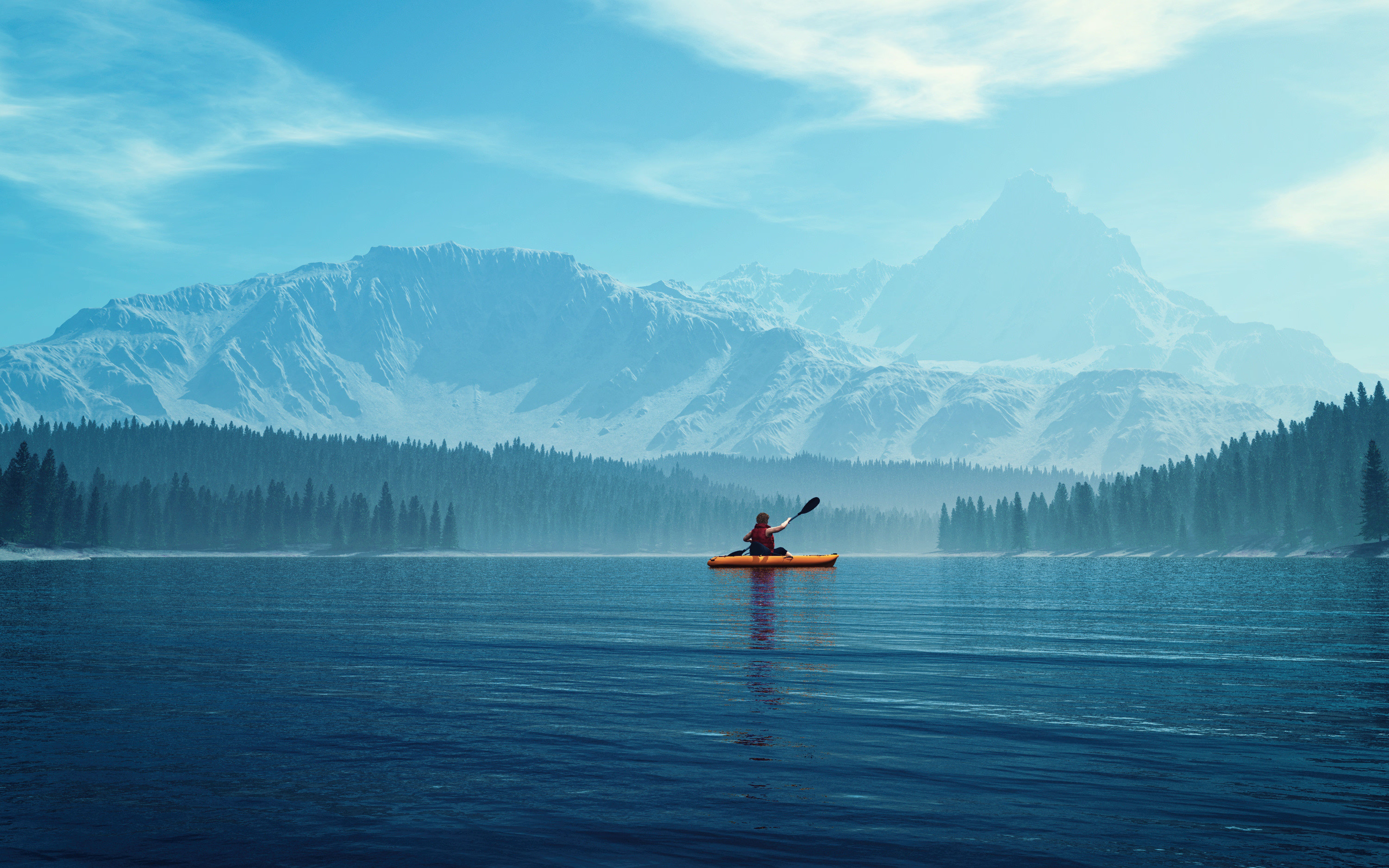